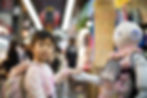
From predicting disease to forecasting stock market trends to determining decision-making for self-driving cars, Machine Learning (ML) and Artificial Intelligence (AI) are pushing forward as technologies of the future. But AI and ML won’t replace the need for human beings in the freight brokerage industry.
Why? The industry's technology infrastructure is not in a place where entire jobs can be replaced. Furthermore, the industry is in a unique space where the skill sets of machines and humans complement each other— AI will replace tasks within jobs that are easily achieved with automation so that humans can focus on tasks that are more well-suited for human decision making.
However, today, we still see a lot of brokerage executives looking to buy AI technology solutions for their businesses without a clear understanding of the sorts of tasks AI can or can’t replace and the components that serve as a foundation for an effective AI implementation.
— Without understanding these, brokerages waste a lot of time, effort, and resources buying the wrong solution, implementing technology in the wrong way, or prematurely implementing a solution.
So, in this post, we clarify what tasks require human touch and management, what tasks are appropriate for full automation, and the prerequisites required for a feasible AI solution. Think strategically about your technology needs first, so when you implement AI-driven solutions, they will assist you to effectively:
Allocate more human effort and energy to customer success, sales, and other back-office tasks.
Automate unnecessary grunt work faster and at scale.
Book more loads and create consistent capacity for carriers.
Expedite new employee on-boarding.
Deliver targeted carrier engagement.
Strengthen carrier and shipper relationships.
Create smarter dynamic carrier profiles.
Effectively forecast supply and demand.
Accurately and dynamically price loads.
— and that’s just the beginning. In effect, you are replicating your best, most productive and experienced broker, and scaling their effectiveness across your entire organization!
Humans Will Always Be the Source of Truth
Look at any industry that is far ahead in terms of automation, such as the self-driving car industry. While people are not driving the car (lower human-touch levels), they are sitting in these cars, monitoring the car’s decision-making with regard to activities such as: turning into the right lane, avoiding pedestrians, and managing unforeseen risks. Human touch is still needed.
Similarly, within a brokerage, there are tasks that simply aren’t suited to automation and will always need a human touch, such as:
Customer success and account management.
Sales management.
Exception management.
Any communication or action that involves empathy and personal understanding.
For example, let’s say a broker is helping a shipper move a load, but their carrier says their driver is going to be late. The customer success representative is going to have to communicate that to the shipper, empathize with them, re-establish trust to the best of their ability, and offer them another useful alternative.
Now, you could use AI to predict (using different data sources such as ELDs and historical behavior) whether the driver is late or going to be late. This empowers the customer success representative to be proactive in their problem-solving. But the actual communication and management of the shipper relationship must come from a human being.
You Don’t Hire Talent to Read Emails All Day Long...
Human knowledge is necessary and fine, but it isn’t scalable and is vulnerable to inaccuracies and churn. For example, same-day spot-freight matching is currently achieved using carrier sales reps who have to sort and sift through 1000’s of emails, disorganized sticky notes, and online load boards. These activities are stressful, inefficient, and are better automated so that carrier sales reps can focus on other tasks that deserve more attention.
Think about it— freight brokerages today are hiring qualified, college-educated employees. Having them spend 90% of their workday reading spreadsheets or emails is a waste of their skillset and talent.
Tasks susceptible to automation are the ones that are repeatable and rely on lots of data to back each decision, such as:
Carrier matching with the right fulfillment opportunities.
Accurate and dynamic pricing and price-forecasting.
Document processing which includes back-office activities such as email capacity extraction and invoicing.
Predict and forecast supply and demand.
Automating the above tasks can help brokers make 1000’s of informed decisions every day. No human being can do these themselves quickly, accurately, in real-time, and at scale. But automating these parts will improve human productivity by augmenting and empowering them within their existing roles.
Foundations of an Intelligent Technology Solution
In a previous post, we talked about the need for comprehensive data infrastructure. In this section, we get a bit more granular and share the other prerequisites needed so you can start to design a feasible technology plan for your brokerage.
1 - Clean Data
Clean data is the most important prerequisite in this massive shift needed to participate in a new AI future. If you don’t have clean, structured data, you can’t use that structured information to accelerate your most important asset: your team. There are 3 essential attributes of good data:
Standardization. For example, a carrier sales rep might enter ‘flatbed” as an equipment type for a particular carrier, and another carrier sales rep might write “fb” — these inconsistencies hurt the ability for data to be used.
Recency. Data is always less useful the older it gets, so if you get a constant stream of data that is new, machine learning models will continuously get better.
Accessibility. Many brokerages have their data siloed off in their Transportation Management Systems or their track and trace systems. However, it is not easily accessible via API to other services that learn off of that data.
2 - Feedback Loops
Feedback loops assist in ensuring that new data is being ingested to make models smarter. For example, Carrier Engagement feedback loops ensure that every time a carrier opens or quotes on a load in real-time, your system will be informed that:
The carrier is interested in these types of shipments.
You can match future similar shipments to this carrier.
— This is just a simple illustrative example.

3 - Technology Infrastructure
When a brokerage is dealing with data surrounding 10,000’s of carriers and loads, they need to start thinking about handling data-at-scale. These data pipelines need technology infrastructure that can:
Process the data so you can run analysis on it.
Run model analysis on the data to reveal a trend or a pattern.
Most technology partners can help you manage this data pipeline and infrastructure. However, maintaining this kind of software on internal hardware is resource-intensive and would require a considerable in-house engineering investment. Therefore, most companies implementing Machine Learning, whether they are a third party technology provider or a broker’s in-house tech team, are running their software on cloud platforms such as AWS, GCP, or Azure.
4 - Feature Generation
What goes into your machine learning algorithm? You need to flesh out which data points are important to the decision-making process. These can all be reverse engineered based on the outcomes you want to achieve. For example, correctly matching shipments to carriers requires data on:
Historical shipments
Lane preferences (user-entered or predicted)
Equipment types
Engagement information (email opens, quotes, etc.)
— And this is just the tip of the iceberg.
5 - Human in the Loop
A machine takes an input and spits out an output. However, you need a human to verify if the models are actually working towards certain measures of success.
For example, your AI software might decide that Carrier A should be matched with Shipment X. But a human being should ultimately judge whether this output is correct or incorrect, validating the model. And if the model’s suggestion is incorrect, a human needs to assess why:
What data is skewing the decision?
What additional data points do we need to add?
What data points do we need to remove?
AI and ML solutions need human input to be optimized; the human will always be the source of truth.
You don’t want to let AI run wild in your brokerage because it won’t work right out of the box. Initially, AI will make mistakes. But as a human being begins to verify every decision and set a baseline for accuracy—machine learning algorithms will improve and become smarter.
It’s important to begin now. The longer a company delays these initiatives, the smarter competitor systems are becoming.
The Symbiosis of Man and Machine
Effective technology solutions require humans and machines working together in a symbiotic relationship. The initial steps to leverage technology solutions for your brokerage are to:
Define your business outcomes.
Clarify the tasks that still require human touch and management.
Clarify the tasks that are appropriate for automation.
Implement all prerequisites needed for any proper AI solution.
— Whether you achieve that using resources within your organization or through a technology partner, be sure to have someone (a champion) that understands the strengths and limitations of AI, and how it pertains to your business needs. Thinking strategically about how technology can augment your business efforts will assist you to build a more competitive and defensible brokerage.